The CLIN31 keynote speaker is dr. Roman Klinger (University of Stuttgart, Germany)
Title: Show-don’t-tell — how emotions are communicated in text and how psychological theories can help us in computational emotion analysis
Abstract: Emotion analysis in text has seen great improvements with well-performing models on data sets for various domains. Unfortunately, these models do not work well across domains; and that is not surprising – we cannot expect a model trained on fairy tales to learn how emotions are communicated in social media. This actually motivates the question how emotions are indeed communicated in various domains, as this might help to better understand the task and potential solutions.
In this presentation, I will therefore discuss how emotions are communicated in social media and literature. This involves the interpretation of events as a main ingredient of everyday communication. To link events and emotions, we will look into psychological theories which indeed explain this link and outline ideas for future computational emotion analysis under consideration of psychological theories.
Short bio
Dr. Roman Klinger is a senior lecturer at the Institute for Natural Language Processing (IMS) at the University of Stuttgart. He studied computer science with a minor in psychology, holds a Ph.D. in computer science from TU Dortmund University (2011), and received the venia legendi in computer science in Stuttgart (2020). Before moving to Stuttgart, he worked at the University of Bielefeld, at the Fraunhofer Institute for Algorithms and Scientific Computing, and the University of Massachussetts Amherst. Roman Klinger’s vision is to enable computers to understand and generate text regarding both factual and non-factual information. This finds application in interdisciplinary research, including biomedical text mining, digital humanities, modelling psychological concepts (like emotions) in language, and social media mining. These topics often constitute novel challenges to existing machine learning methods. Therefore, he and his group also contribute to the fields of probabilistic and deep machine learning.
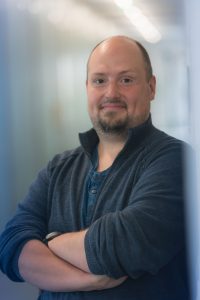